Welcome back to Currents, a monthly column from Reimagine Energy dedicated to the latest news at the intersection of AI & Energy. Every last week of the month, I send out an expert-curated summary of the most relevant updates from the sector. The focus is on major industry news, published scientific articles, a recap of the month’s posts from Reimagine Energy, and a dedicated job board.
1. Industry news
Trump is back: what does this mean for Energy and AI?
Donald Trump’s re-election was arguably November’s most globally significant news. But what does this mean for the energy and AI landscape? Trump recently vowed to “dramatically increase baseload power” to slash electricity costs, prevent brownouts, and “WIN the battle for AI superiority.” How will this play out?
Key figures in his administration suggest that the focus will be on fossil fuels over renewables. Chris Wright, an oil and gas CEO who claims “there’s no climate crisis,” has been nominated to lead the Department of Energy. North Dakota Governor Doug Burgum is set to head the National Energy Council, which will be granted sweeping authority over federal agencies to establish American “energy dominance” around the world.
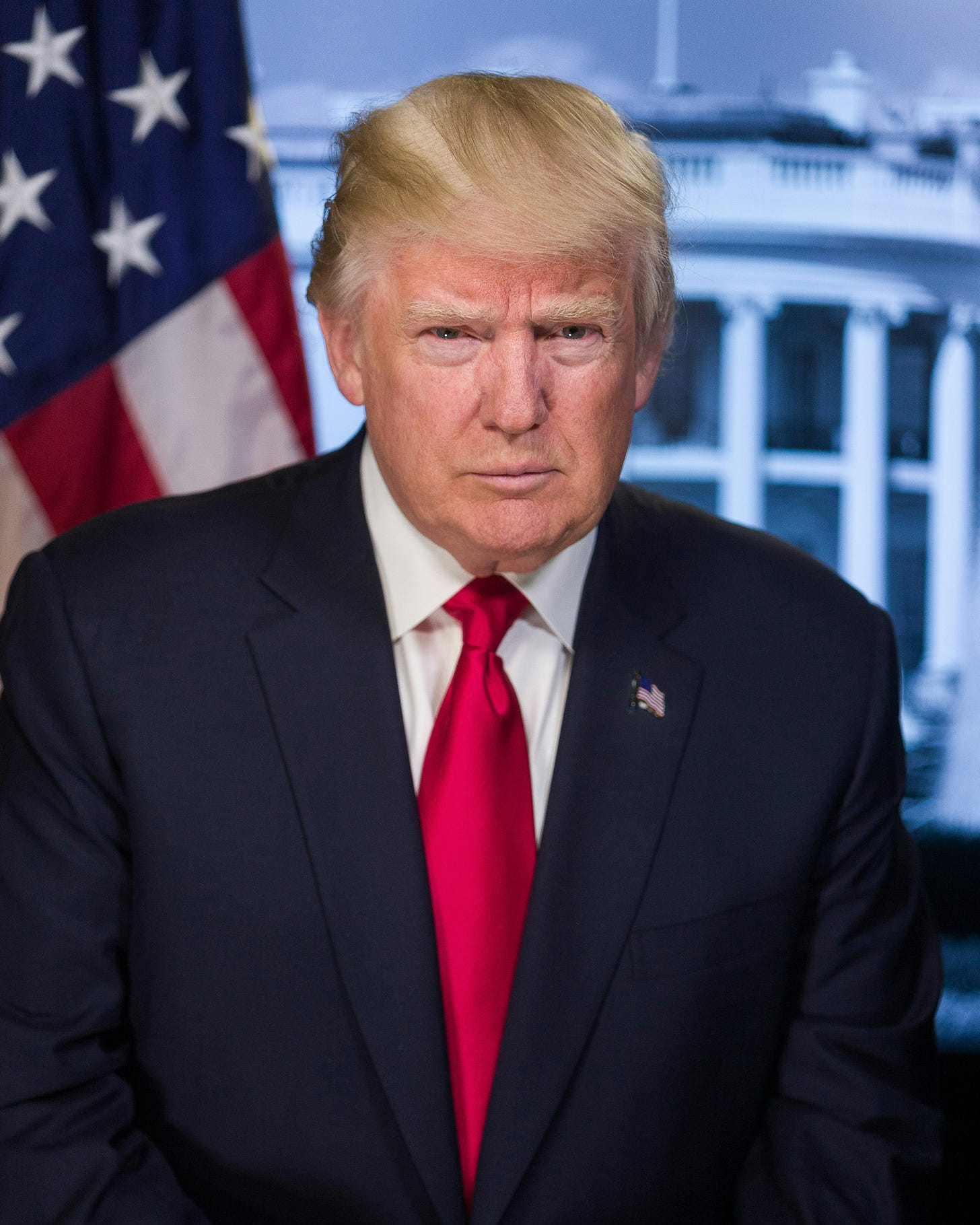
Yet, the landscape is more complex now than during Trump’s first term. ExxonMobil CEO Darren Woods stated that the U.S. should not pull out of the Paris Agreement, claiming this could hinder Exxon’s investments in carbon capture, hydrogen fuel, and EV battery technology—initiatives that rely on federal tax credits established under the Inflation Reduction Act (IRA). Repealing the IRA altogether won’t be straightforward for the Trump administration. Many Republican districts stand to gain from its investments, making a full rollback politically tricky.
What I’m thinking: The last thing we need is to politicize decarbonization efforts. Climate change is a global challenge and requires collective effort, not ideological battles. Instead of getting bogged down in rhetoric and polarized discourse, we should improve policies based on what’s effective. Now more than ever, we need to focus on solutions that make immediate economic sense—those are the ones the market will adopt regardless of political winds.
Trump’s re-election is likely to have a significant impact on U.S. and global energy policy, but it won’t stop economically sensible solutions. As the smart folks at WattCarbon observed: “We may witness a shift from top-down to bottom-up decarbonization, driven by grassroots movements rather than federal mandates. […] With limited funds, maximizing the impact of every dollar spent on decarbonization becomes crucial.”
Europe’s industrial struggles and AI-driven decarbonization
While the U.S. is grappling with policy shifts, Europe faces its own energy challenges. Swedish battery maker Northvolt has filed for Chapter 11 bankruptcy, entering a restructuring phase to stay afloat. This is a blow to Europe’s ambitions in energy storage—a critical component for electrifying transportation and balancing renewable energy supply and demand.
Germany is also navigating a difficult situation. Early November brought a “Dunkelflaute”, a period of minimal wind and sunlight, leading to a spike in electricity prices and increased reliance on fossil fuels. While the grid remained stable, electricity costs and carbon footprints rose sharply. This added to existing doubts about the nation’s Energiewende efforts and comes amid political instability. German Chancellor Olaf Scholz’s coalition government collapsed amid disputes over economic recovery and the energy transition. With the finance minister sacked and snap elections set for February, crucial policies now hang in the balance—including the 2025 budget, without which many climate and industry support programs could stall.
Amid these challenges, the German startup scene has been notably active, with three companies raising capital to improve energy efficiency through AI:
Predium, a Munich-based PropTech company, raised €13 million in Series A funding. They use AI, satellite imagery, and 3D modeling to help property owners assess ESG risks and prioritize renovations for maximum cost savings and subsidies.
Juna.ai, a Berlin startup, secured $7.5 million to develop AI systems that make industrial manufacturing self-learning and adaptive. Their technology optimizes processes in real-time, improving efficiency and reducing energy consumption and emissions.
KUGU, another German PropTech firm, raised €8.5 million in Series A funding. They offer products that optimize and control energy systems, helping real estate companies meet emissions targets. KUGU manages over 12,000 buildings and serves major housing companies, utilities, and metering service providers across Germany.
What I’m thinking: Despite the hurdles of a continent struggling from an industrial and deep-tech manufacturing standpoint, Europe’s software push for bottom-up decarbonization offers hope. Multiple software startups leveraging AI to improve energy efficiency and reduce operating costs demonstrate that innovation doesn’t wait for political stability. Similar to the U.S., the way to navigate these moments of policy uncertainty is to focus on economically viable products, rather than betting solely on technologies that depend on rebates and subsidies.
Other projects and reports that caught my attention
I enjoyed DeepMind’s latest report “A new golden age of discovery”, which provides an overview of how AI is transforming scientific disciplines. The report emphasizes AI’s potential to tackle many challenges in the energy sector as well, such as creating simulations to advance fusion energy research or improve weather forecasting. I also appreciated the discussion around creativity. LLMs are trained to minimize anomalies, and I’ve noticed this in my own experience. I rely on LLMs as my “sparring partners” when debating ideas and exploring different points of view, but I rarely use them during the idea generation phase—the output is usually bland. We definitely shouldn’t rely solely on LLMs to define the areas toward which we conduct our research, at least for now.
I also looked at FlexiDAO’s report on granular meter data accessibility. I talk a lot about the importance of granular energy data and how, without it, AI-powered solutions can’t optimize our energy systems effectively. The report is comprehensive, and the different map visualizations provide a clear way to understand which markets founders in this space should focus on and which emerging markets are on the rise.
Finally, I explored Robin Hawkes’ GB Renewables Map. I found this through a LinkedIn post about how a brand-new 1.1 GW wind farm in the UK has been “off” nearly 70% of the time during 2024 due to limitations on the transmission system between Scotland and England. The analysis led me to Robin’s GB Renewables Map, and it was really fun to explore—I suggest you check it out!
What I’m thinking: I love that every month brings exciting new products, reports, whitepapers, and interactive platforms that open new doors and unlock new connections. There’s so much to explore, with new developments showing up every day. On the other hand, the abundance of possibilities and shiny new tools can be distracting and even generate anxiety. As professionals in this sector, we need to master the ability to discern the trivial many from the vital few. Understanding that not all efforts are equal and that some activities yield exponentially higher results than others is crucial for prioritizing effectively.
2. Scientific publications
Large Language Models for the Creation and Use of Semantic Ontologies in Buildings: Requirements and Challenges. This paper studies how Large Language Models (LLMs) can aid in constructing and querying semantic models in the building domain, specifically using the Brick schema. Semantic ontologies like Brick offer a standardized, machine-readable framework for representing complex building systems, but creating and querying these models is challenging and often requires specialized expertise. The paper explores how LLMs can address these challenges by lowering the expertise barrier and making the creation and use of semantic models more accessible.
What I’m thinking: I feel we haven’t seen enough LLM applications in the buildings industry compared to other fields, so I’m glad that research like this is starting to emerge. At the same time, I would have liked to see a more practical application of these ideas. The paper is quite theoretical, and it would have been helpful to see how this approach could work in the reality of building operations and management. Hopefully the next step!
Boosted Conformal Prediction Intervals. This study introduces a boosted conformal procedure designed to improve conformal prediction intervals by targeting specific properties like enhanced conditional coverage or reduced interval length. Through gradient boosting, the authors systematically refine a predefined conformity score function without altering the underlying trained model. This approach operates post-training, relying solely on model predictions. Experiments demonstrate that starting from conventional conformal methods, the boosted procedure achieves substantial improvements in reducing interval length and decreasing deviation from target conditional coverage.
What I’m thinking: This paper is math-heavy and isn’t directly focused on the energy sector, but I found it particularly interesting. Gradient boosting is a powerful tool, and the ability to provide accurate confidence intervals for predictions is valuable across all industries, including ours. In the context of Measurement and Verification of energy efficiency savings, for example, being able to calculate precise confidence intervals for estimated savings enhances the reliability of our results. This method could potentially improve how we assess and communicate the uncertainty in our energy savings estimates, leading to better decision-making and increased trust from stakeholders.
3. Reimagine Energy publications
If you didn’t already, make sure to check out my Python tutorial to predict a full-year of energy efficiency savings when only 6 months of data are available:
4. AI in Energy job board
This space is dedicated to job posts in the sector that caught my attention during the last month. I have no affiliation with any of them, I’m just looking to help readers connect with relevant jobs in the market.
Data Scientist at WattTime
Data Scientist and Machine Learning Engineer - Energy and Food at Eurecat
Senior Data Scientist at Danfoss
Principal Research Scientist - AI for Power Systems at Siemens Energy
Postdoctoral researcher: Combining AI, adaptive control, and digital twins to optimize autonomous systems at Zurich University of Applied Sciences
Conclusion
With so much going on in the sector, it’s not easy to follow everything. If you’re aware of anything that seems relevant and should be included in Currents (job posts, scientific articles, relevant industry events, etc.), please reply to this email or reach out on LinkedIn and I’ll be happy to consider them for inclusion!